The Faces in Things Dataset
We introduce an annotated dataset of five thousand human labeled pareidolic face images, called ``Faces in Things''. Faces in Things is derived from the LAION-5B dataset and annotated for key face attributes and bounding boxes
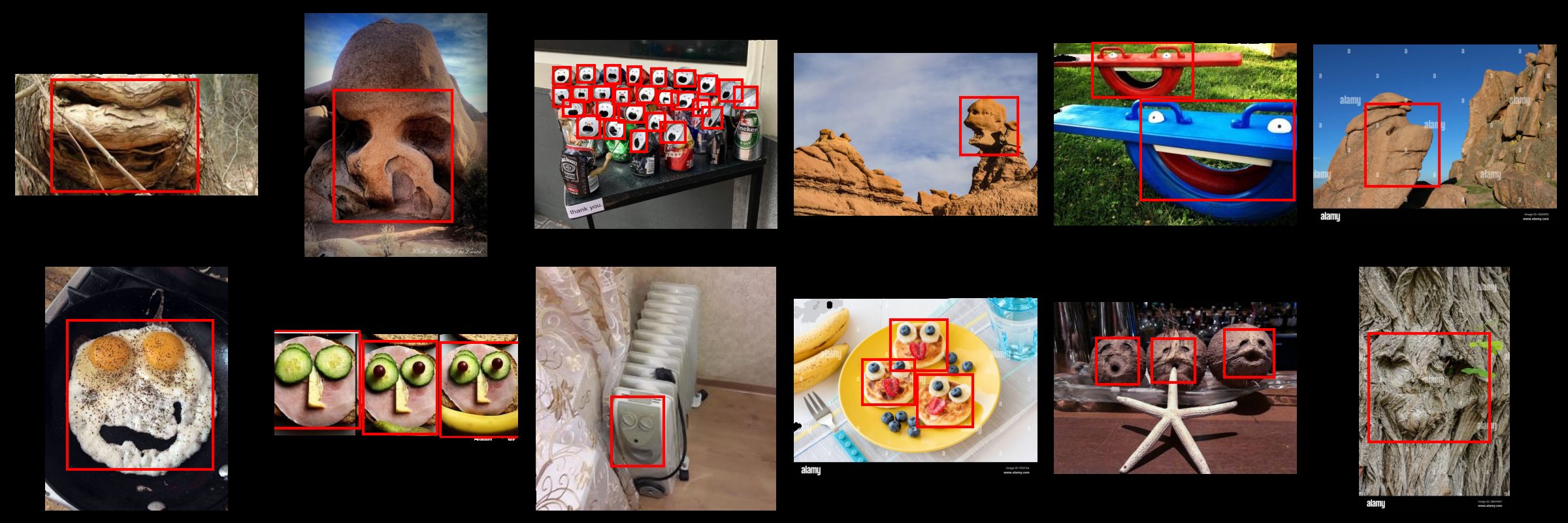